Natural Language Processing: The Importance of Standardised Data
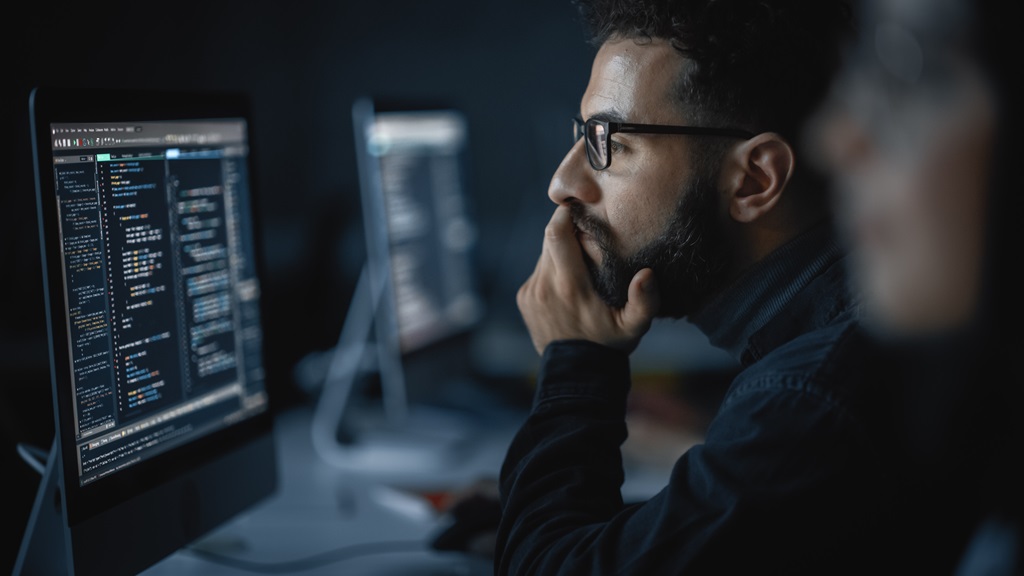
Natural Language processing or NLP dates back to the 1940s when Alan Turing published a research paper named "Computing Machinery and Intelligence" where he mentioned his infamous Turing Test as a way of measuring intelligence. After 1950s most of the NLP revolved around symbolic NLP which is processing text using pre-given set of rules and according to these set of rules machines can do or take certain actions. One of the biggest usages of symbolic NLP can be found with early question answering models where set of questions and answers along with predefined rules, used as a way of automating basic conversations, Eliza ( 1960s) is one of the earliest examples of Q/A applications where it can produce almost human like responses for presented questions using NLP.In the late 1980s, a shift occurred in the field of natural language processing (NLP). Scientists and researchers began exploring the realm of statistical NLP, marking a departure from the rule-based approaches dominant at the time. This new approach utilised statistical models to analyse and understand language, paving the way for advancements in tasks like machine translation, text classification, and speech recognition, which remain essential components of NLP technology even today.

Image 1 : Eliza Prototype in 1962
Building upon the foundation laid by rule-based approaches in the 1950-1970s and statistical NLP in the late 1980s, the field of NLP transformed significantly in the 2000s. One main reason for this explosive growth is thanks to the World Wide Web and the vast amount of digital data it generated. Wide popularity of the internet and IT systems forced most of the data to be transformed into digital formats. The filling up of a void which previous NLP data scientists had. Availability of digital text data provided the fuel for training increasingly sophisticated machine learning models, leading to breakthroughs in areas like deep learning and neural networks. These advancements significantly enhanced NLP capabilities, allowing for tasks like sentiment analysis, automatic text summarisation, and chatbot development to reach new levels of accuracy and complexity. As a result, NLP entered a new age, capable of not only understanding language but also interacting in human-like ways.
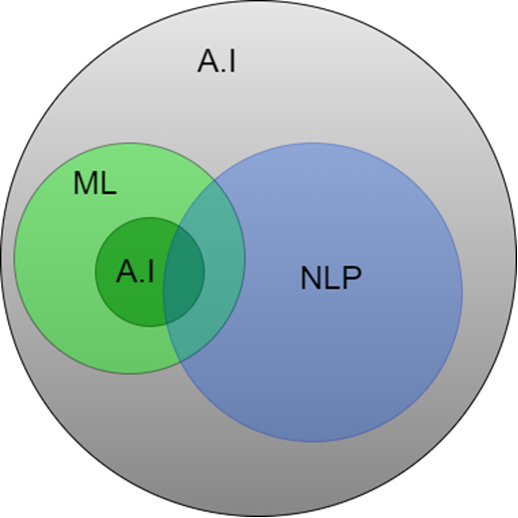
Image 2 : A.I machine learning and NLP
Modern NLP boasts a diverse set of capabilities, each contributing to various business domains. Following are some key tasks and their impact:
- Machine translation: Breaks down language barriers, enabling seamless communication and collaboration across diverse cultures, fostering international trade and business partnerships.
- Text classification: Automates categorising large amounts of text data, aiding businesses in managing customer feedback, streamlining document processing, and optimising targeted marketing campaigns.
- Sentiment analysis: Uncovers the underlying emotions and opinions buried within text data, empowering businesses to understand customer sentiment, improve product offerings, and personalise customer service interactions.
- Automatic text summarisation: Condenses large volumes of text into concise summaries, saving valuable time for busy professionals and facilitating efficient information retrieval in various fields, from legal documents to scientific research.
- Chatbot development: Enables businesses to create virtual assistants that engage in conversations with customers, providing 24/7 support, answering FAQs, and automating repetitive tasks, ultimately enhancing customer experience and reducing operational costs.
- Speech recognition: Transforms spoken language into text, fostering greater accessibility for individuals with disabilities and powering innovative applications like voice search and voice-enabled smart devices, leading to a more convenient and hands-free user experience.
- Entity extraction: Automatically identifies and extracts specific pieces of information from text data, such as names, locations, dates, and organisations. This capability allows for automating tasks like data entry, information retrieval, and knowledge base population, significantly reducing manual effort and increasing the accuracy and efficiency of various business processes. Imagine automatically extracting customer information from support tickets, product details from online reviews, or financial data from reports, all without human intervention. This is the power of entity extraction in action.

Image 3 : NLP Extracting Features from Raw Data.
These tasks are great examples of transformative potential of modern NLP, paving the way for advancements in numerous business domains, from marketing and customer service to healthcare and education.
Availability of digital data in the early 2000s undoubtedly ignited a revolution in NLP technologies. However, the future holds even greater potential as the focus shifts towards the standardisation of data across similar platforms and within specific domains. When data is organised using consistent formats and labeling conventions, NLP models can be trained more efficiently on larger and more comprehensive datasets. This data standardisation opens doors for more powerful NLP systems with the capacity to achieve the following:
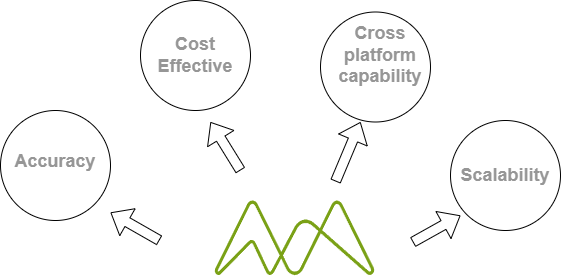
Image 4 : How Structured Data can be Useful.
Improved accuracy: Standardised data minimises errors and inconsistencies in the training data, leading to more reliable and precise NLP tasks like sentiment analysis, which can offer more accurate insights into customer feedback or product reviews.specially in banking applications and customer servicing apps.
Enhanced cross-platform compatibility: Standardised data allows NLP models developed in one domain, like healthcare, to be more easily adapted and applied to others, like finance, by reducing the need for significant data re-formatting and retraining, ultimately promoting wider adoption of NLP solutions.
Reduced development costs: Data standardisation decreases the need for time-consuming tasks like data cleaning and formatting, thus freeing up valuable resources for model development and innovation. This leads to faster development cycles and the ability to explore new NLP applications across various industries.
Facilitated knowledge transfer: Standardised datasets encourage collaboration and knowledge sharing within the NLP community. With consistent data formats, researchers can compare and share their findings more easily, leading to accelerated advancements in the field as a whole.
Conclusion
In essence, standardised data paves the way for NLP technologies to 'take off' with unprecedented capabilities and wider applications. With the hype surrounding A.I. reaching new heights and investments pouring in, it's incredibly interesting to see how the future will unfold. Will A.I. live up to the extraordinary expectations, or will it face unforeseen challenges on the path to transforming our world? Only time will tell, but one thing's for sure: the journey promises to be fascinating and filled with groundbreaking advancements.
From the Blog
Let’s Get In Touch
All information provided is completely confidential and will not be shared with any third party.